In brief
- Three-quarters of the banking industry already uses AI. And despite Gartner's prediction that GenAI will take years to become a key banking trend, recent developments point to an increasing desire toward bringing GenAI into the mix immediately
- Modern technology isn't about finding the perfect tool. It's about picking a tool and using it in the right way — finding the right business case and then using and losing the technology. Tech advances so quickly that it’s become disposable, and your next solution will likely work for a couple of years at most
- By implementing fraud analysis on the z16 mainframe using Hogan, Umbrella.ai and IBM’s Telum chip, 100% of bank transactions can be run across deep learning models in real-time, reducing fraud at the average tier-1 bank to the tune of $120 million a year
Why the big rush to apply AI and ML in banking?
Especially given the implications for business. For instance, the primary purpose of AI in banking is to automate tasks and generate predictions using machine learning (ML) models (including complex deep learning). This requires massive computing power and datasets, as well as significant investment.
Also, financial institutions are already major AI users. They rely on cloud-based ML services (e.g., AWS, Microsoft Azure, Google ML) and (mainly) private or mainframe hybrid cloud environments.
There seems to be a new tech development almost every day, and understandably, everyone wants to use it immediately. But there’s much to be gained by assessing what you need to do realistically and which solutions can help you achieve that. And by ensuring that you’re using what you already have to its greatest effect — getting the biggest bang for your buck, so to speak — before splashing out.
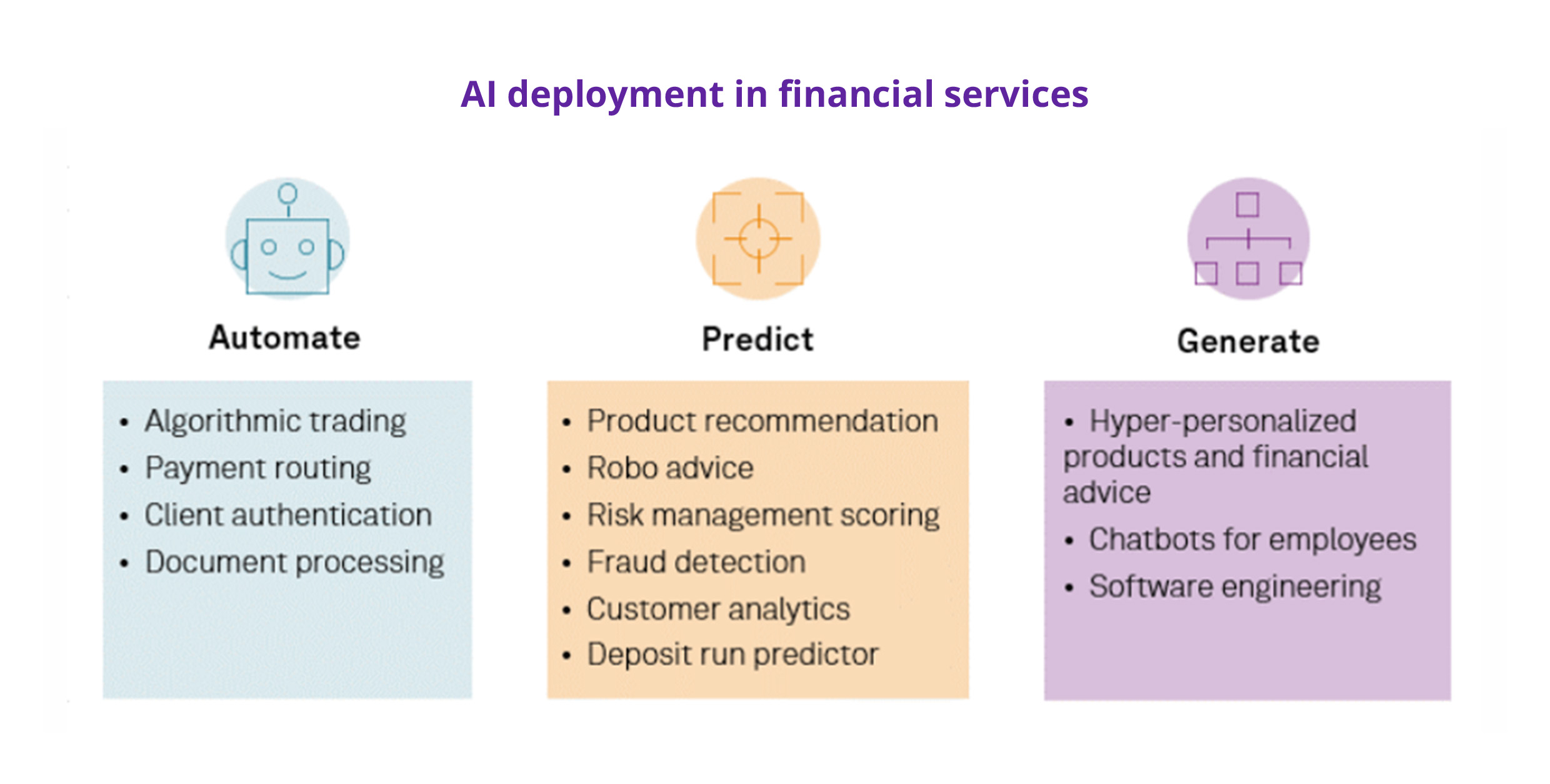
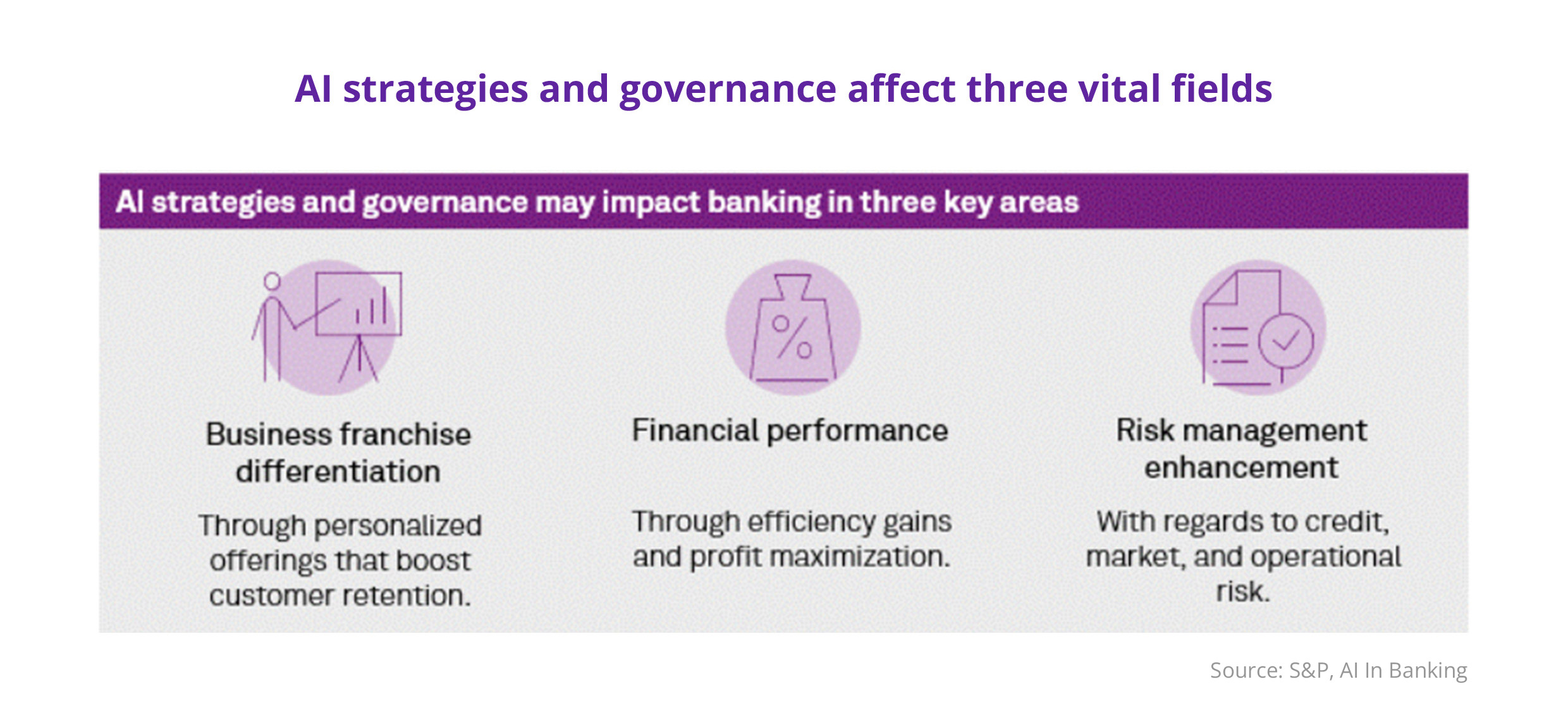
Source: S&P, AI In Banking
So, where are we now?
Three-quarters of financial institutions already use AI and recent developments signpost an increasing desire toward bringing GenAI into the tech mix as soon as possible. GenAI’s low-code/no-code software could provide significant cost savings too, including cutting operational costs and risk linked to outdated infrastructure and labor-intensive processes.
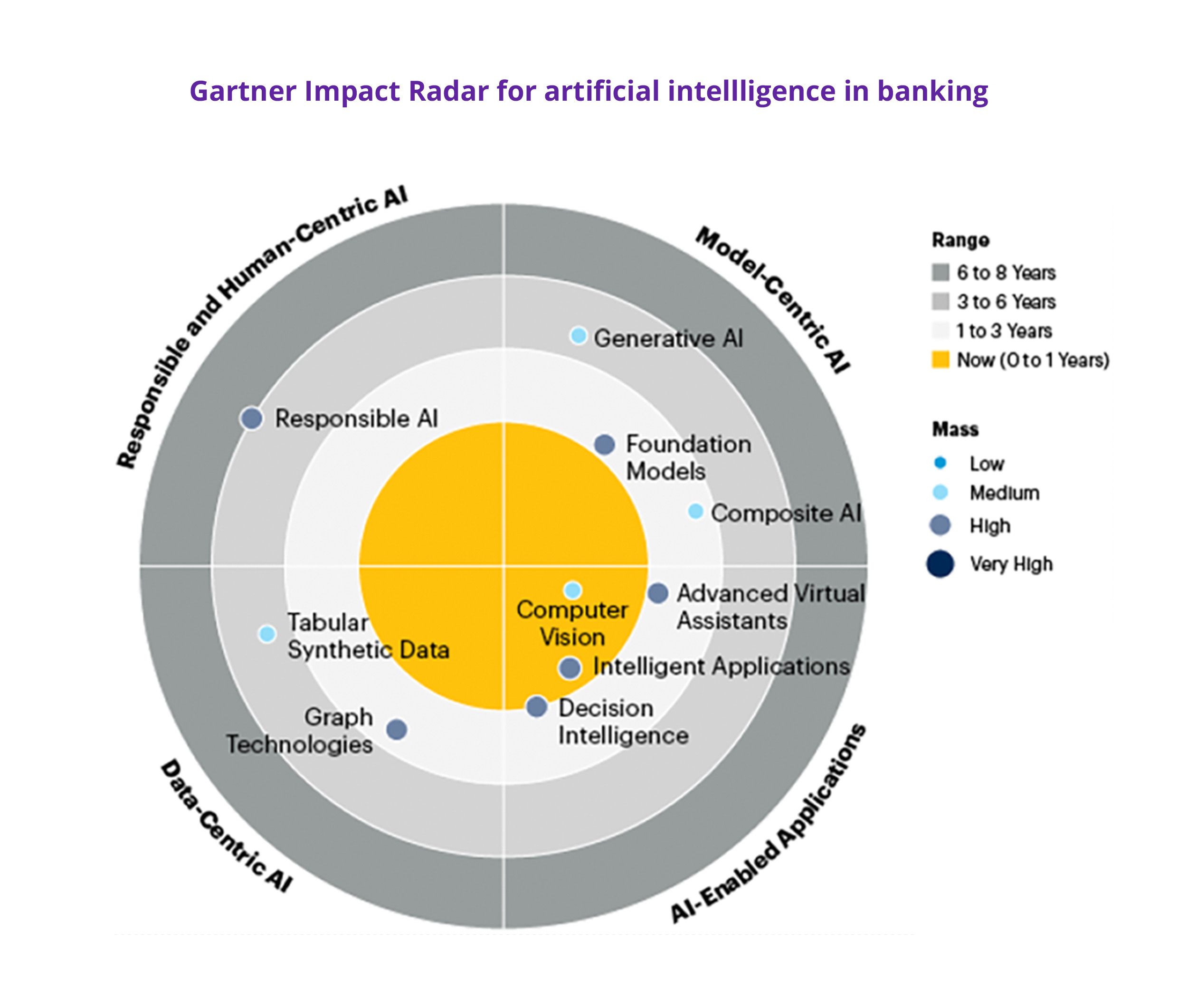
Source: Gartner Impact Radar 2023
The main AI trends in banking, according to Gartner:
- Computer vision: Enhance operational efficiency in KYC, AML, ID verification, fraud detection and underwriting
- Decision intelligence and graph technologies: Upgrade front- to back-office applications
- Core AI technologies: Heighten accuracy and applicability in marketing and risk management via foundation models. Generative adversarial networks (GANs) are widely used for generating realistic images, videos and voice recordings that are often indistinguishable from authentic data
- Model-centric AI: Introduce encouraging developments such as composite AI and GenAI to support fresh initiatives
- Data-centric AI: Focus on data analysis for ethics, accuracy and explainability
- AI-enabled applications and use cases: Expand work on conversational user interfaces, smart spaces, robots, etc.
- Responsible and human-centric AI: Double down on ethical AI development, risk management and beneficial social impact
Credit where credit’s due
Improving decision-making, streamlining operations and using product hyper-personalization to raise client returns could significantly boost your operating revenues and reduce costs. S&P projects that a 10% reduction in bank staff costs could increase return-on-equity by about 100 basis points and cost-to-income ratios by about 3% (based on the S&P Global Ratings' Global Top 200 rated banks).
AI strategies can potentially provide competitive advantages to banks with the capacity and flexibility to make the best use of them.
While upscaling risk management, AI could incidentally affect the perception of bank risk profiles. Banks that can accurately price credit risk by employing patterns buried in the data to figure out the likelihood of customer debt repayment will enhance their workout models, reduce troublesome loans and optimize accuracy.
It’s possible to use AI to improve risk management principles in several ways. However, that won’t stop inferior implementation from generating reputational and operational risks and a negative risk position for the bank.
Get it right. Partner with an experienced technology provider who knows the banking ropes.
New EU regulation initiative
Europe’s new AI Act has established a comprehensive regulation for AI products and services within a risk- and use-case-driven environment. The Act includes responsibilities for foundation models supporting general-purpose AI systems. Similar moves are afoot in other countries like Canada, Brazil, Chile and the Philippines.
In the United States, the National Institute of Standards and Technology (NIST) has established an AI Safety Consortium. Its aim is to work with industry to develop new standards, including sector-specific guidance driven by risk management. As well as governmental regulatory activity, there’s an increasing acknowledgment of industry’s duty to protect society by initiating or strengthening AI governance.
The new AI regulation application is based on what risk users will likely introduce and which activities should be regulated. The EU has considered four kinds of risk:

“Minimal” and “limited” risks signify that AI is used for basic internal tasks like routine automation. They exclude interaction with real users and customers (e.g., bots or work related to the knowledge base). Minimal risk is not highly regulated, and most of the regulation to be applied is around explicitly informing customers that they’re interacting with bots.
“High” and “unacceptable” risks cover AI systems that guide decision-makers. This includes query scoring, things related to client data and working with clients in general. These AI applications will be highly regulated, with many rules to follow closely when using these types of AI systems. Activities related to AI systems that voluntarily or involuntarily affect users by changing free will or behaviors will be banned altogether.
So, AI systems that influence high-risk users, such as seniors and minors, or how society functions, like social scoring and biometric screening, and then analyze the data with the implicit involvement of customers — where they’re from, what they’re doing, and so on — will be affected.
Regulations introduced in 2024 and enforced by 2026 will mostly apply to high-risk systems.
Financial services
AI regulation for financial services will determine access. In financial services, AI systems analyze client data for credit scoring and fraud detection.
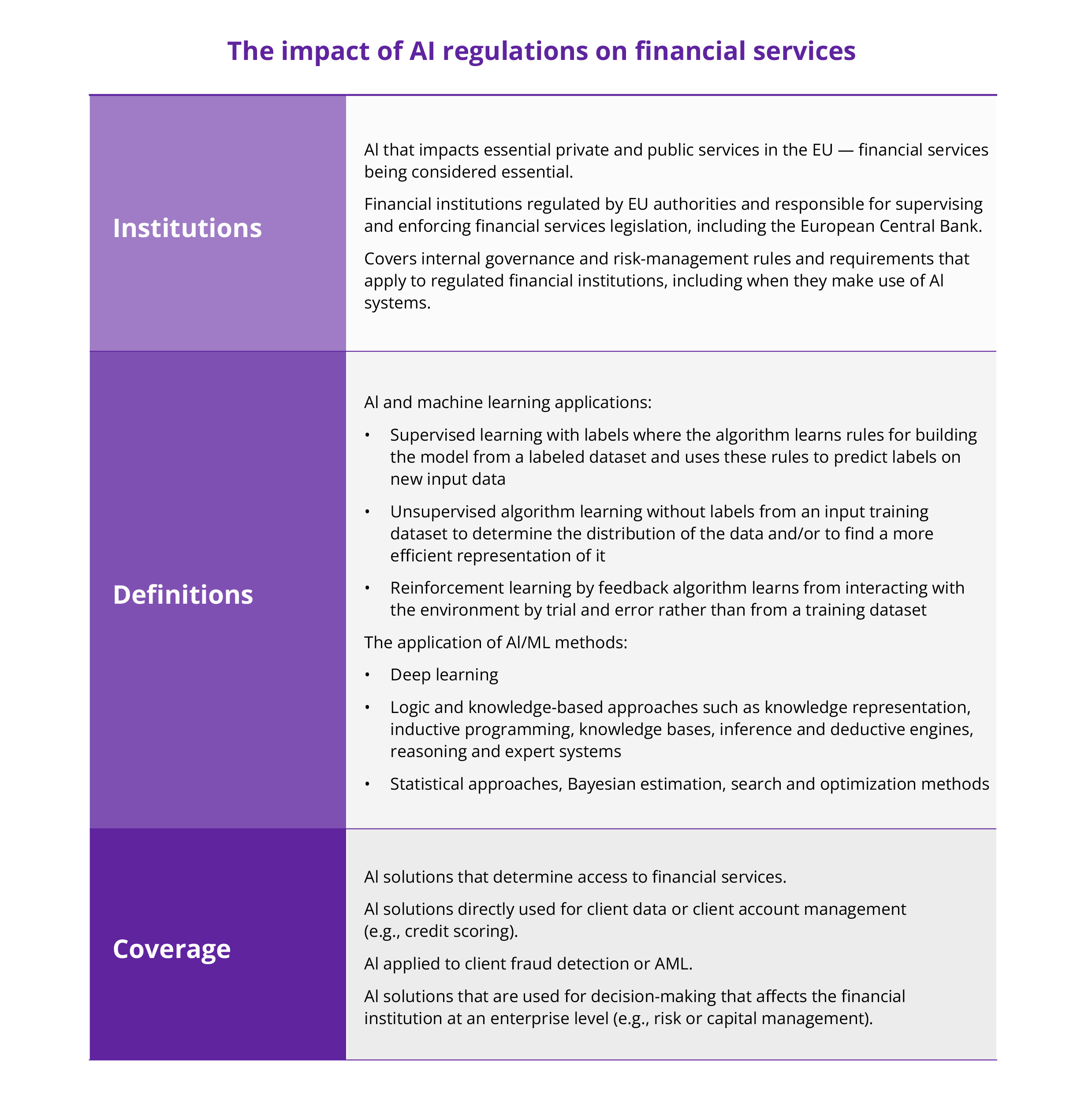
Understanding the potential security risks of AI
From an analytics perspective, we’re in an exponential growth phase of this remarkable technological age. Consequently, virtually anything built 2 years ago is already out of date. And the question is, how does a company use outmoded technology in a controlled, non-toxic way, mindful of the knock-on effects of the slightest error?
Take ChatGPT, for example. No one knows where the app’s information originates (or its bias/copyright/integrity). Yet more and more employees are encouraged to use the app to produce corporate blogs, articles, white papers and even lines of code. No wonder financial institutions are considering building ringfenced AI services (an extension of the ongoing company debate: “Do we go to the cloud or stay on-prem?”).
This is a critical issue because, for effective AI and sophisticated analytics, you must be sure that your data integrity is on point. That being so, it makes sense to work on-prem, insulated from the internet. Your corporate large language model (LLM) or grammar-checking app can be trained exclusively on the company’s internal documentation (it will never leave the mainframe). In fact, the most secure solution flies in the face of contemporary IT thinking, which advocates sending everything to the cloud.
The Hogan x core banking system
As a processor and system of record, Zoreza Global’s Hogan core banking platform has become the driving force behind some of the world’s most influential banks.
Now, we’ve enhanced Hogan’s exceptional mainframe hybrid cloud solution by taking the best IBM Z solutions and providing blueprints for banks with aging cores to be “migrated to platform.” Hogan x can even help you create a whole new digital bank.
The platform follows the BIAN (banking industry architecture network) principles of being componentized and composable. It takes advantage of Z Linux and other containerized and cloud-native solutions. And Zoreza Global can also deliver Hogan x in consumption-based, as-a-Service models.
Find your best fit
Mainframe hybrid cloud architecture allows you to adopt a best-fit strategy for application implementation. Here’s a banking application ecosystem example (across IBM Z and cloud).

Source: IBM
The applications are divided into three distinct areas: Digital channels for client engagement, operational processing (e.g., order management, marketing and sales) and core transactions and data (core banking and credit cards). These applications must work together to share real-time information and maximize their business effectiveness. Integration is vital for achieving wholesale interoperability.
A mainframe hybrid cloud model with IBM zSystems helps clients optimize costs, performance and agility based on their application type and best-fit infrastructure. Take digital channels, for example. A high degree of omnichannel interaction relies on partner and customer information integration to create the best possible customer experience. This, plus variable change and workloads, makes hybrid cloud-based solutions your perfect choice.
Environmental, social, and governance (ESG)
An often-overlooked advantage of the mainframe hybrid cloud architecture is environmental sustainability. Combining agile cloud working with energy-efficient mainframes enables banks to optimize their data center footprint and energy consumption (data centers use around 1% of the world’s electricity), contributing to a greener planet.
Consolidating Linux workloads on five IBM z16 systems instead of running them on compared x86 servers under similar conditions can reduce energy consumption by 75%, space by 50% and the CO2e footprint by over 850 metric tons annually.
A strategic banking imperative
However, mainframe hybrid cloud architecture in banking is not just a technical choice. It's a strategic imperative, balancing the need for security, reliability and scalability with the agility and innovation demanded by digital working. By integrating mainframe resilience with AI, ML and the flexibility of cloud computing, banks can remain competitive, compliant and customer-centric. Success relies on using this to maximum effect, ensuring that banks not only master current challenges but are also primed and ready to exploit future opportunities.
As technology evolves, AI and mainframe hybrid cloud strategies will play a pivotal role in banking’s digital transformation, mapping a journey that pairs tradition with innovation, stability with agility and performance with efficiency. Now is not the time to hesitate.
This is no time for “wait and see”
A colleague recently attended a prominent conference where a speaker touched on this very problem. “We don't know where technology’s going, so we're going to kind of wait and see where it comes out,” he said. The trouble is, they won’t get to see where it comes out because, given tech’s exponential growth, progress never stands still long enough to be rationalized.
The fact is, banks need to get on board with foresight and open minds if they’re going to apply AI rapidly, securely and profitably.
Informed collaboration is essential
That’s why Zoreza Global’s support is so valuable. Deploying optical character recognition (OCR) is a great example. For one client, we offered up four tools that read documents saying, “It doesn't really matter which one you pick. In 2 years, it'll be out of date.” The procurement department told us to look at some others, too, turning it into a full-scale exercise to try and pick the perfect tool.
But modern technology selection isn't about drilling down to the right tool. It's about picking and using a tool correctly, finding the right business case, then using and losing the technology. Tech advances so quickly that it’s become disposable — planned obsolescence is built in, and your next solution will likely work for a couple of years at most. So, rather than making a 5+ year investment in a z16 solution, refresh your AI technology every 2 years. In other words, start planning for its replacement as soon as you sign the procurement contract.
Modernize mission-critical processes like fraud detection
Business challenge: AI is often applied outside the Z platform, which requires data to migrate over networks. This is expensive and insecure and reduces your ability to score transactions for risk.
Business impact: The IBM Z AI on-chip accelerator enables Hogan and related zLinux workloads to run real-time AI capabilities on the mainframe where the data currently exists. This improves AI performance, security and cost. Also, activities like automated credit decision-making and loan modification can be done directly on the Z platform, making AI insights and business decisions quicker and easier to assimilate. Celent estimates that if 100% of transactions were screened, each bank could save in the region of $100 million.
Save millions with Umbrella.ai
AI is deployed for a variety of use cases, such as credit scoring, fraud and AML. However, while the bulk of banking and payment transactions execute on mainframe platforms, the AI detection is often off-platform (less than 10% of transactions go through an Al inferencing model in real-time due to latency, cost and customer friction issues). This is why so many fraudulent transactions go unmonitored and undetected.
Zoreza Global’s Umbrella.ai can save you millions in fraud detection with on-mainframe inferencing. The key component in this is Umbrella. Over 35 years, the Umbrella application architecture has become a proven technical foundation designed to provide a rapid integrated development environment (IDE) on z/OS. Umbrella is used by more than 40 tier-1 financial institutions globally, facilitating around 100 applications per bank. That's thousands of applications in mainframe development.
Umbrella.ai prevents fraud
In partnership with IBM, Zoreza Global is reducing the time it takes to score or analyze a transaction. This gives clients the chance to check virtually 100% of their transactions and minimize time/cash wastage. Umbrella.ai goals include:
- Scoring 100% of incoming transactions while meeting existing SLAs
- Leveraging existing fraud models or deploying custom models
- Reducing scoring latency by implementing fraud prevention natively on z/OS alongside the card authorization system
- Leveraging the MLz COBOL scoring service to:
- Greatly simplify the scoring call-out
- Reduce overhead to invoke the scoring service
Revolutionary fraud detection and risk management
One of ML’s major plus points is the escalation of fraud detection. Traditional rule-based systems are inflexible and unable to adapt quickly to developing fraud patterns. Meanwhile, ML algorithms continuously learn from transactional data, identifying fraudulent activities faster and more accurately.
Umbrella.ai and ML accelerate fraud detection and prevention
Real-time identification of fraudulent transactions, actionable insights at scale and increased fraud prediction accuracy are all possible with AI/ML on IBM Z.
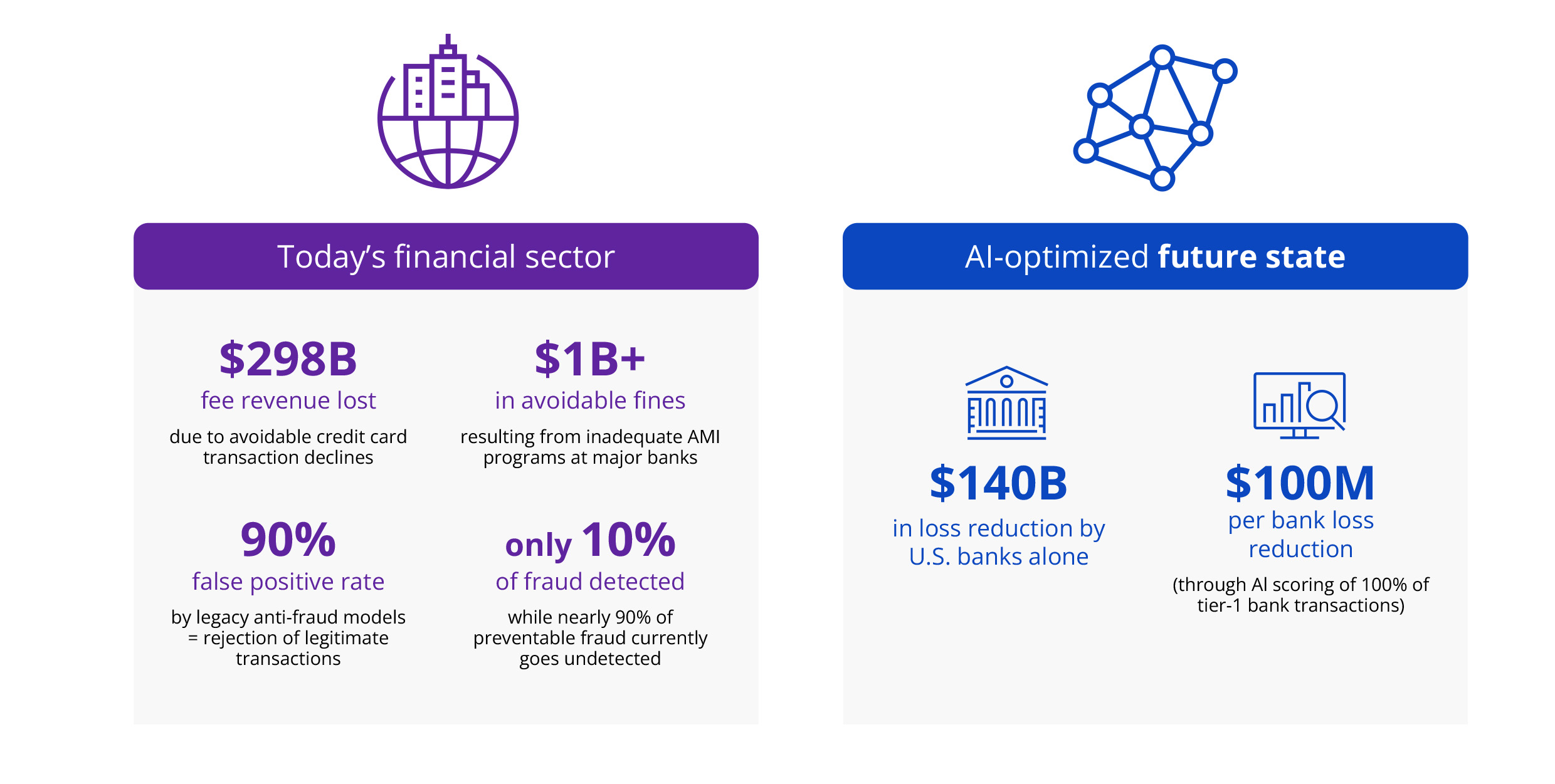
Source: IBM
The increased accuracy of deep learning models enables them to cut excessive false positive rates substantially. This means banks can pass transactions through fraud detection without impacting the customer experience or hemorrhaging money.
By implementing fraud analysis on the mainframe using Umbrella.ai and IBM’s Telum chip, 100% of your transactions can be run across deep learning models in real-time, reducing fraud at the average tier-1 bank by $120 million a year.
Umbrella architecture, IBM z16 and AI agility
The partnership of Umbrella with AI and the IBM z16 delivers impressive business insights (no need for data science skills).
Around 70% of financial transactions are run on IBM zSystems and that’s also great news from an ESG standpoint — 40% less energy is consumed during inferencing on the mainframe than running on some kind of server farm.
- Power any IBM z/OS application with Al-enhanced SQL and Umbrella.ai
- Uncover and monetize insights hidden in your data
- Identify similarities, differences and correlations
- Get interpretability out of the box
- Apply a single model across multiple questions
- Minimize Al deployment complexity
- Improve false negatives with deep learning models
The IBM z16 supports the most popular ML algorithms, providing clients with an Al cloak to help them improve processes and drive greater business value from their existing investments.
Time to talk
Can you afford to stand by while competitors build a handsome lead and enduring advantage?
Discover how to best apply AI and ML to get the most bang for your buck. Also, learn how Hogan-powered mainframe hybrid cloud enablement can help you become a better fraud detector and prepare for future threats and opportunities. Visit our website. Or, if you have a specific AI, GenAI or ML issue you need to get off your chest, contact us.