In brief
- Predictive analytics is a business intelligence technology that can predict events, outcomes or behaviors based on historical and real-time data. Predictive analytics tools determine the most probable scenarios by discovering patterns in available data using statistical modeling, data mining and machine learning
- Grouping items into segments based on data patterns allows insurance claim analytics to automatically identify potentially high-cost complex claims and match them with more experienced adjusters. The processing of simpler low-cost claims can be expedited with automation tools. So, the adjustment becomes more efficient, speeding up both standard and complex claims processing
- Predictive modeling in insurance can also inform management of the likely risks and outcomes of specific decisions — assessing the potential impact of external factors like climate events or inflation on sales, for example. It can also deliver cash flow and revenue projections, which can inform proactive risk management, focusing on mitigation rather than damage control
Data analytics is a technology that insurers can no longer afford to overlook. That’s why 67% of insurers expected an increase in spending on data analytics in 2022, according to Deloitte’s report. Of those 67%, 20% estimated the increase would be significant for their companies.
Insurance data analytics comes in many forms, and predictive analytics is one of them. It is the technological equivalent of a crystal ball for insurance companies, albeit more reliable. Using it, insurers can accurately predict outcomes to drive growth, improve customer experiences, and accelerate processes like risk assessment and underwriting.
We at Zoreza Global, a DXC Technology Company, help clients in the insurance and other industries to unlock the full potential of siloed data with advanced analytics. Today, let us share our experience in analytics solutions and break down what predictive analytics is, how it can benefit insurers, and how it can transform their operations.
What is predictive analytics?
Predictive analytics is a business intelligence technology that can predict events, outcomes, or behaviors based on historical and real-time data. Predictive analytics tools determine the most probable scenarios by discovering patterns in available data using statistical modeling, data mining, and machine learning.
This technology is one of the major applications of machine learning (ML) and deep learning, the two offsprings of artificial intelligence (AI). It’s also associated with data science and big data technologies.
Predictive analytics algorithms can be trained to accomplish a variety of tasks across all industries, such as:
- Assessing risks
- Determining staffing needs
- Forecasting cash flows
- Predicting customer churn
- Identifying cost-saving and efficiency-improving opportunities
How does predictive analytics work?
While there are multiple types of predictive analytics models, introducing the technology itself into business processes is a six-step undertaking:
- Identifying the problem and setting goals. First, the team behind the predictive analytics solution should clearly understand the problem to solve and the objectives to attain.
- Collecting and organizing data. All raw data should be centralized in a single place, such as a data warehouse system. It can be pulled there manually or automatically.
- Cleaning raw data. A quality data set has to contain only standardized, unique, and complete entries. To prepare it for predictive modeling, all duplicates and incomplete and irrelevant entries should be removed.
- Developing predictive models. This is where data analytics specialists choose the most suitable modeling techniques and train the algorithms to identify patterns using the available data set.
- Testing and deploying the models. Before the model rolls out enterprise-wide, it has to be tested in various scenarios and fine-tuned to avoid inaccurate conclusions or unexpected behavior.
- Monitoring performance. Specialists should keep an eye on the deployed predictive analytics models to weed out potential inaccuracies and inefficiencies, which could result from changes in patterns, data, etc.
Six benefits of implementing predictive analytics in insurance
The exact benefits of introducing predictive analytics in insurance depend on the chosen use case, software stack, and implementation quality. That said, in general, this technology can enable insurers to reap some of the following benefits:
- More accurate fraud detection. Fraud doesn’t have to remain a cost of doing business in insurance. Predictive analytics can automatically flag suspicious behavior during underwriting and claim processing, reducing losses from both hard and soft fraud.
- Improved customer experience (CX) and retention. Predictive analytics in insurance can power omnichannel personalization and identify customers at risk of cancellation. Speeding up processes like underwriting contributes to a better CX overall, too.
- Automated tasks and accelerated processes. Predictive analytics can streamline risk assessment, pricing calculation for custom policies, and claim processing from the first notice of loss (FNOL) to settlement.
- Optimized costs. Reduced fraud, partially or fully automated risk assessment workflows, and improved customer retention all reflect on the bottom line—in a good way.
- Data-driven decision-making. Predictive analytics excels at forecasting cash flows, sales, customer churn, and more, enabling management at all levels to make well-informed strategic and tactical decisions.
- New products and services. Predictive analytics enables usage-based insurance and preventative maintenance, as well as personalization at scale.
Three challenges to introducing predictive analytics
Adding predictive analytics tools to the software stack isn’t without its challenges. Here are the three top challenges of introducing advanced analytics insurance companies deal with, according to Deloitte:
- Legacy system dependence. For an average insurer, the median age of legacy estate reaches 18 years. This poses significant compatibility issues, as integrating modern predictive analytics solutions may become next to impossible. The cumbersome legacy estate can also exacerbate data silos and fragmentation. Modern technology solutions however enable insurers to deploy AI solutions onto legacy systems.
- Implementation complexity. Introducing predictive analytics solutions may require legacy system modernization and a unified data management strategy implementation first. Those are massive undertakings on their own, but early solutions can be deployed to accelerate the benefits.
- The technology may be considered not mature enough. While it is already dubbed the next step in data analytics evolution, some executives perceive it as not being mature enough. The technology may employ black-box models, meaning algorithms don’t show their work. That’s why introducing predictive analytics still requires embedded governance.
Nine use cases for predictive analytics in insurance
Insurance data analytics finds its application in every aspect of business operations, from underwriting to claims management and customer experience. Here are nine ways this technology changes insurance operations for the better.
Assessing risks in underwriting
In insurance, risk analysis is everything when it comes to underwriting. Accurately predicting risks for a specific customer optimizes premiums and prevents losses for the company.
Traditionally, assessing risks has been a mostly manual process, with a few basic metrics like age or occupation taken into consideration. However, today’s advanced risk analytics in insurance can automatically deliver a risk score based on dozens or hundreds of metrics, informing underwriting, pricing, policy coverage, and more.
For example, predictive analytics in health insurance can identify how likely a prospective policyholder is to turn to medical services and how costly those interventions can be. To do so, it can take into account a variety of parameters, from age and gender to preexisting conditions, lifestyle, and daily habits.
Detecting fraud
Fraudulent claims cost around $80 billion a year to US-based P&C (property and casualty) insurers, according to one estimate. Another assessment puts the total cost of insurance fraud at approximately $300 billion in the United States. And a 2022 report demonstrated that adjusters suspect fraud in 20% of claims.
So, it’s no wonder why fraud detection is among the main uses of advanced data analytics for insurance companies. According to the 2022 Insurance Fraud Report, 42% of insurers opted for predictive analytics to weed out fraudulent claims.
To do so, predictive models can be trained to identify and flag suspicious behavior from the first notice of loss (FNOL) to approval or denial. Once trained, they can detect subtle patterns associated with common and sophisticated hard and soft fraud schemes. In those cases, they should trigger a manual review.
Accelerating claim processing
Predictive analytics excels at grouping items into segments based on patterns in the data. In claim processing, this allows insurance claims analytics to automatically identify potentially high-cost and complex claims and match them with more experienced adjusters. At the same time, low-cost, simpler claim processing can be expedited with automation tools.
As a result, the adjustment becomes more efficient, speeding up claim processing for both complex and standard claims.
It doesn’t only reflect well on the bottom line. Prompt claim resolution is one of the four key drivers of a positive claim experience. So, customers are more likely to be satisfied with their provider that streamlined its claim processing with predictive analytics.
Preventing claims
Predictive analytics is the cornerstone of the preventative maintenance approach in insurance. This approach means that insurance companies aim to help policyholders avoid such an event instead of handling claims when an insured event occurs.
To make preventative maintenance possible, predictive analytics has to be coupled with the Internet of Things (IoT). IoT refers to a network of connected devices tracking specific metrics and transmitting them over the internet.
Armed with that data, predictive models can forecast when industrial equipment, vehicles, and home infrastructure like electrical wiring are likely to break down and diagnose potential issues.
For example, predictive analytics in property and casualty insurance can continuously analyze the home fire alarm system and notify the policyholder when it’s time to change it. Or, it can track vehicle sensor data and inform the car owner when they should check their brakes or tires.
Predicting customer churn
Across industries, over half of consumers would leave a company after several bad experiences, according to a 2022 PwC survey. In insurance, the risk of customer churn is serious in 18% of negative reviews.
Predictive analytics can help insurance companies identify that risk at scale: a feat impossible even for an expert team analyzing customer data 24/7. The technology can do so by analyzing behavioral patterns on a microscopic level and across a variety of channels.
For example, combined with other technologies like natural language processing, predictive analytics can spot negative reviews on social media. Or, it can raise alarms if the policyholder looks into contract termination within the mobile app.
Personalizing customer experiences at scale
When it comes to customer relationship management, insurtech data analytics can do a lot more than just predict churn. As the technology excels at finding patterns in swaths of data, it can easily create detailed policyholder segments based on their past behavior and future needs and intents.
Predictive analytics also powers the next level of personalization: personalization at scale. This concept refers to analyzing customer data in real time to anticipate their needs. Based on those predictions, personalization at scale solutions then deliver valuable, individualized customer experiences across multiple channels and touchpoints.
To unlock the full potential of customer analytics in insurance for upselling, cross-selling, and personalized offers, the insurer can combine third-party data with first- and zero-party information. For example, if an existing policyholder has planned a trip, this data can prompt personalization-at-scale tools to suggest travel insurance to them.
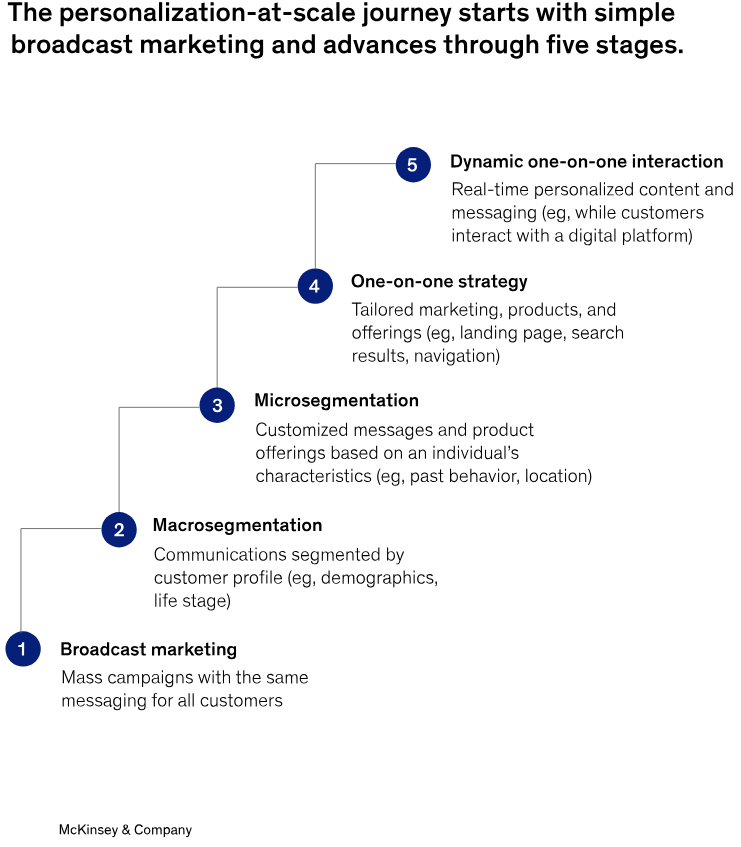
Source: https://www.mckinsey.com
Streamlining underwriting
As mentioned above, predictive analytics automates risk assessment based on dozens or hundreds of behavioral and demographic factors. It streamlines the most time-consuming part of underwriting.
So, instead of an employee promising to get back to the customer within 24 hours with a quote, this quote can be automatically calculated online or in a mobile app.
Accelerated underwriting improves both the customer experience and employee satisfaction. Insurance agents and brokers can focus on the more value-added aspects of their jobs, contributing to a better customer experience.
What’s more, predictive analytics can streamline fraud detection during underwriting. To that end, the technology can be set to identify a risk of misrepresentation or suspicious behavior and automatically trigger a manual review when necessary.
Powering new insurance products
Insurers no longer need to stick to a rigid range of packages and policies. Services, terms, and coverage options can be combined into offers tailored to every customer.
Predictive analytics can then calculate risks for every personalized offer—all within seconds and without human involvement.
What’s more, predictive analytics models can assess data in real time to continuously adjust pricing. For example, in car insurance, predictive analytics can ingest driver behavioral data from IoT sensors (e.g., speeding, hard braking, usual routes, etc.) and adjust the premiums according to changes in risk.
Premiums and insurance products continuously adapting to the customer’s individual behavior are known as usage-based insurance. It comes with two key benefits:
- Continuous risk assessment allows for accurately matching premiums with risks, optimizing pricing and costs in the process
- Offering coverage at lower costs for low-risk consumers helps insurers compete in a world of declining purchasing power
Implementing proactive risk management
Risk assessment doesn’t have to be applied only to underwriting and pricing. Predictive modeling in insurance can inform C-level management of the likely risks and outcomes of certain decisions, as well.
For example, predictive modeling can be used to assess the potential impact of external factors like climate events or inflation on sales. It can also deliver cash flow and revenue projections based on historical and real-time internal and third-party data.
All of this can inform proactive risk management. It’s the idea of concentrating on preventing losses by mitigating risks instead of reacting to existing threats by doing damage control.
Ready to unlock the power of predictive analytics in insurance?
Predictive analytics can accelerate or fully automate underwriting, pave the way for new insurance products, and deliver highly personalized customer experiences at scale. All of that can help insurers gain a competitive edge, optimize costs, improve customer retention, and more.
However, implementing predictive analytics in insurance isn’t without challenges. We’d be honored to help you overcome them without a hitch. Contact us to kickstart a conversation on how we can enable you to extract maximum value from predictive analytics solutions.