In brief
- Decision-making is a critical aspect of business success, and it is essential to have a clear understanding of the decision-making process and the factors that influence it.
- Leveraging advanced analytics technology and machine learning can help organizations make more informed decisions by providing insights and identifying patterns that may not be immediately apparent.
- Effective decision-making also requires strong leadership, clear communication, and a willingness to consider different perspectives and opinions.
What’s the one thing that separates top managers from the contenders?
Great decision-making
Some say it’s an art, some say it’s a science. However, one thing we can all agree on, great decision-making is the defining characteristic of innovative executives; leaders who regularly influence company and colleagues in a positive way.
So, how do they do it?
All decisions should be based on data, but not just any old data. It has to be the right data, in the right place, at the right time. And that means fine-tuning our “data metabolism”, but we’ll come to that in due course.
New technology + more data ≠ better decisions
Predictive analytics are used to support decision-making for most functions of a capital markets organization. However, while firms are forging ahead, modernizing technology and integrating data, their decision-making abilities are losing traction. This is because organizations struggle to cope with too much data, with not enough data and, especially, with not having the right type of data for the particular decision that they’re trying to make.
Executives are encouraged to focus on the primary KPIs for their business — whether that’s revenue, risk, compliance or innovation — to the exclusion of most other business variables. Meanwhile, employees are often unable to add significant value in strategic decision-making, because the whole operational culture is too segmented to cascade the data necessary for meaningful outcomes. Modernizing data analytics creates a new avenue for decision-making, with comprehensive data sets and the ability to look beyond a handful of favored KPIs.
Business and IT need to work together
The business wants:
- Not just user-friendly technology. They want technology that delivers business value
- Easy access to real-time data with reliable quality on a consistent basis
- A unified interface that serves multiple needs, integrating access to repositories containing data definitions, lineage, data requirements and system inventories
- More granular, traceable and frequently collected data for statutory and regulatory reports
Keep IT response:
- Centralize operational data-management activities while sharing responsibility to enhance data controls
- Cloud data technologies, AI and cognitive tools that have a serious impact on operations, risk management and data-management initiatives
- Tools to enhance analytics capabilities in areas like data governance, metadata management, data lineage, data quality, issue management, master and reference data management, data modeling, and the reporting of data operations and monitoring
Analytically challenged banks
Faced with troubled economic times, investment banks and all other financial actors have been pressed into rethinking products and services, and improving quality through data analytics.
This is more challenging for capital markets firms because of the breadth of data and data sources that can be included in the analytics process. Here are seven categories of analytics use cases that are employed across the full range of capital markets players:
Analytics are used to support decision-making in most functions of a capital markets organization
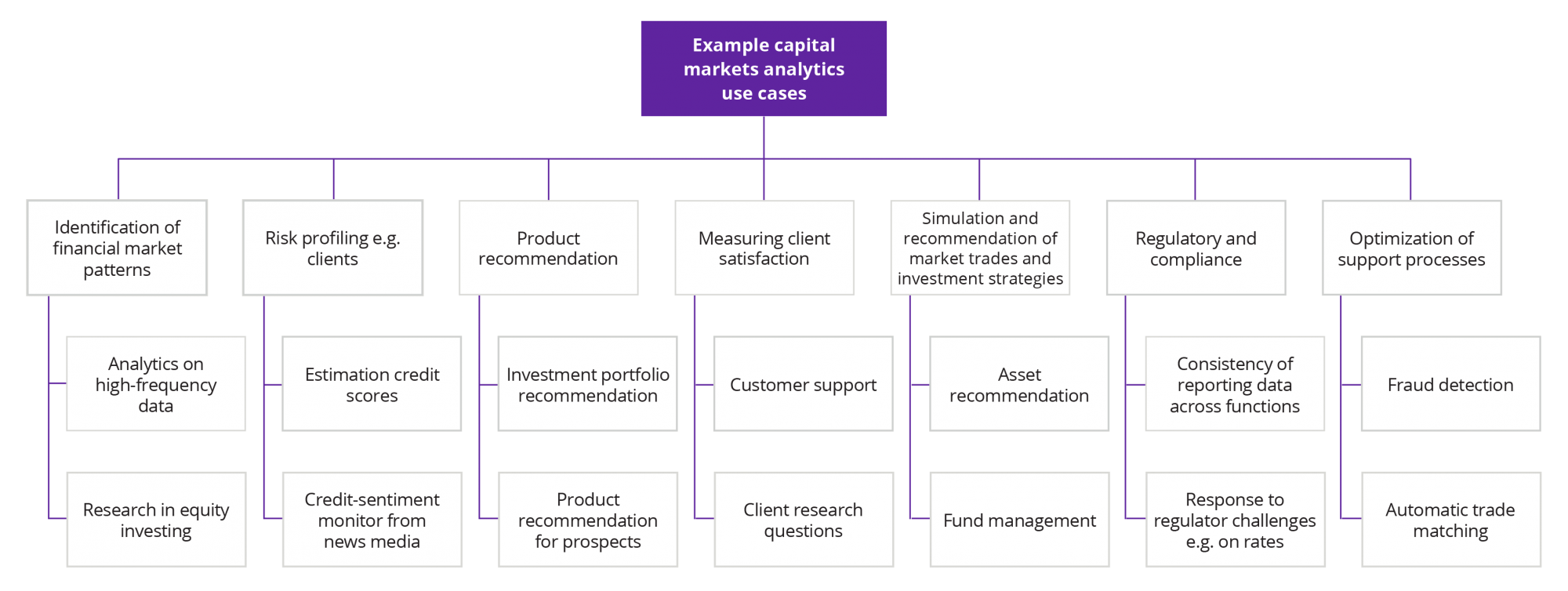
Evolving analytics technology
The data analytics technology landscape is vast and subject to fads. As a result, organizations often have an uneven distribution of analytics technologies across their business units. Paul Hewitt has designed a data evolution model to create a structured approach to maximize the use of data analytics assets.

This model takes a broad view of data analytics assets to include the technology, the process and the data itself. For example, bringing in new technology — like a data lakehouse — doesn’t mean an organization has automatically evolved. To deliver value from this investment, first, you need a new governance model to get the best out of your new and incredibly powerful storage analytics technology. Then you can upgrade skill sets and build a set of processes to help with decision-making.
Using the evolutionary model correctly allows organizations to build sound data analytics capabilities. Then they can devolve these capabilities from the pioneering units to other parts of the bank. The benefits of a mature, devolved data analytics approach are clear. In 2021, an Economist (UK) survey reported that 61% of executive respondents claimed they’d had to cancel a digital project because they lacked the right data.
The state of decision-making in capital markets
However, it’s not just about data analytics. Our researchers looked into the process of business decision-making, identifying the three key stages — discover, develop and defend. “Discover” is the low-risk, experimentation phase and requires data variety to widen the search landscape. In the “develop” state, data comes at you faster and, therefore, velocity is your ally. The “defend” state is all about volume and protecting the investments you’ve made.


Develop: Portfolio strategy, sales, relationship management, portfolio construction and rebalancing, leadership
Discover: Research and analysis, fund and portfolio performance, marketing, client reporting, risk reporting
Defend: Asset servicing, fund accounting, performance attribution, trade control, regulatory and compliance
Improving suboptimal leadership
That's the idealized approach. In the real world, though, while employees focus on innovation, operations and compliance, most executives are focusing on strategy, growth and client relationships, etc. But the team’s competing in three areas, and if executive attention is on permanent “divert”, the company is left with suboptimal decision-making around the “develop” state and an inability to scale up.
It’s like when the CFO takes over as CEO; they need to switch perspectives or run the risk of leading the business from a “defend” perspective, exclusively, missing out “develop” altogether. Which brings to mind the wise old saying, “Never let an accountant run your football club”. In less sporty language, executing “discover, develop and defend” decisions demands that top execs delegate “discover” and “defend”, so they can prioritize forward-looking creative initiatives and focus on “develop”.
Capital markets are behind the curve
Three data drawbacks are hindering capital markets firms

First, even with the best reengineering, most organizations’ business processes date from the 1990s. Which means they’re applying over 20-year-old structures to unfeasibly higher data volume, variety and velocity. Today’s play is all about customer-centric processes that enable users to access organizational services through their smart devices.
Second, while data can increase in value over time, generally, it decreases in value even though organizations burn through large sums of money storing, maintaining and managing it. In fact, data can become toxic if it’s not supposed to be stored or kept outside of regulatory compliance needs. Information wealth has value in exchange, not storage.
Capital wealth has value in storage, increasing in worth over time (subject to interest rates, of course). The disparity between the life cycles of data and capital wealth value is a disconnect. Therefore, all our management and control structures for balancing risk and reward no longer work effectively.
Third, a combination of social media, mobility and analytics has led customers, partners and employees to become “appified” (expecting instant gratification via their smart phones at next-to-no cost). And as consumers exhibit increasing appification, organizations are forced to analyze and make insight-based decisions in a much tighter timeframe.
Decisions, decisions, decisions
We believe our approach to data-analytics evolution has a deep impact on improving an organization’s data metabolism.


Lines of business professionals need to make decisions. The firm has the technology which is developed and improved over time, but there's a massive gap between the decisions they want to make and the analytics the technology solutions churn out. Part of that is because there are different types of decisions being made. Data sets are being pulled off in droves but no one actually knows which answer they're looking for or which data they really need to pull in. Now, that takes experimentation and a shift of practice.
By creating an AI-powered organization, we can move from highly customized experimentation loops to rapid experiments. In essence, there's a technology improvement happening and, with the emerging practice, we change the way we work because we have created a pervasive analytics environment.
Keep decision-making up to speed
Every time you advance the technology and process around analytics solutions, you have to balance that with an equal effort to make sure that your decision-making function is capable of exploiting your ecosystem upgrade. Or put another way, improvements in working out what type of decision you’re dealing with and which decision state it’s in, need to equal the development and maturity of the data analytics that underpin it.

With the correct approach, organizations with ready access to virtually limitless amounts of data will be able to use the data to make decisions at the speed appified customers demand. This is the desired state for mature data metabolism.
What is data metabolism?
Data metabolism is the ability to deliver relevant data and insights at the right time and right speed to the right people, to optimize decision-making. It explains an organization’s struggle to process its data effectively, improving decision-making while evolving data engineering.
Companies with a healthy data metabolism roll out thousands of new products every week, with a design-to-production cycle time of less than a week. If an organization can’t do that, it’s falling behind.

Over the last decade, the data maxim has been “big is brilliant, but bigger is better”. Concepts such as big data and data lakes were seen as key enablers for organizations becoming data-driven. But all they did was perpetuate the “organizational paralysis in decision-making” problem. Then future investments in data, analytics and information management tools, not only failed to generate positive returns but also caused further dysfunction. This is why the foundations of a highly functioning data metabolism are built using a mature data-analytics ecosystem.
Quick-fire results
Our research into next-generation operating models during the early stages of the pandemic, showed one repeating pattern emerging. Organizations were making decisions in weeks rather than months or years. We heard of long-time stalled decisions being actioned within weeks. Similarly, as experts set out to understand what it really means to be a data-driven organization, executives talked about low-level satisfaction with their data initiatives and programs.
Organizations complain about too much data and not enough data but, particularly, about not having the right type of data for the kind of decision that they’re looking to make.
So, we concluded that the problem around data wasn’t about access but about the maturity of data use. Organizations need to drive their technology and people to become a data-driven and AI-powered enterprise.
Fine tuning your data metabolism could make a world of difference to profitability and business outcomes in general.
Get in touch
You can take a deeper dive into data metabolism and making better decisions by downloading Khal’s earlier white paper, “How to supercharge your data metabolism”, here.
If you’d like to find out what Zoreza Global, DXC and realigning your data metabolism could do for your organization, visit luxoft.com/capital-markets or contact at financialservices@luxoft.com.